Artificial intelligence is a new technical discipline that studies and develops theories, methods, technologies, and application systems for imitating human intelligence extension and expansion.
Artificial intelligence research aims to enable machines to execute some complex jobs that would otherwise require intelligent humans.
That is, we hope that the machine will be able to solve some difficult jobs for us, not simply monotonous mechanical activities, but also ones that demand human wisdom.
In this article we will be diving deep into the 6 fields of AI and other branches of artificial intelligence. But first of all, what is artificial intelligence and component of AI.
What Is Artificial Intelligence (AI)?
Artificial intelligence (AI) involves using computers to do things that traditionally require human intelligence.
AI can process large amounts of data in ways that humans cannot. The goal for AI is to be able to do things like recognize patterns, make decisions, and judge like humans.
Artificial intelligence (AI) makes it possible for machines to learn from experience, adjust to new inputs and perform human-like tasks.
Most AI examples that you hear about today – from chess-playing computers to self-driving cars – rely heavily on deep learning and natural language processing.
Artificial intelligence is the simulation of human intelligence processes by machines, especially computer systems.
Specific applications of AI include expert systems, natural language processing, speech recognition and machine vision.
The Artificial Intelligence is a field of engineering that uses highly advanced techniques and technologies for designing softwares and robots to think and act intelligently. It is labeled as artificial because it is the opposite of human natural intelligence.
These systems and machines can replicate the human mind's learning, reasoning, and problem-solving abilities to varying degrees.
Self-driving vehicles are increasingly becoming a reality. The operating system that enables this type of amazing technology starts with software that connects to each of the sensors in the car.
These sensors communicate with and perceive the surrounding environment. For the car to successfully reach its destination, it must continually interact with traffic lights, other vehicles, highways, buildings, and even weather conditions.
Whether it involves self-driving cars, speech recognition, or complex learning techniques, AI — including each of the various expert systems — works seamlessly together
Helpful Content: 10 Practical Uses of AI in Everyday Life
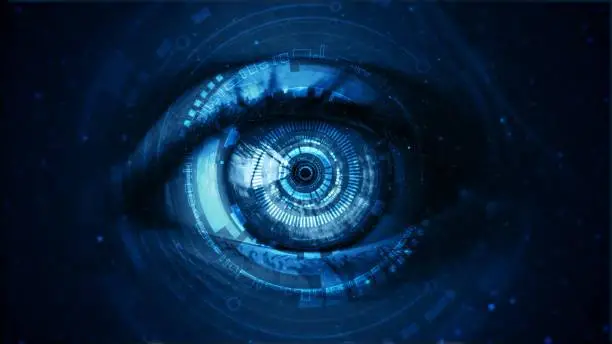
Component of Artificial Intelligence
1. Learning
Similar to humans, computer programs also learn in different manners. Talking of AI, learning by this platform is further segregated into a varied number of forms. One of the essential components of ai, learning for AI includes the trial-and-error method.
The solution keeps on solving problems until it comes across the right results. This way, the program keeps a note of all the moves that gave positive results and stores it in its database to use the next time the computer is given the same problem.
The learning component of AI includes memorizing individual items like different solutions to problems, vocabulary, foreign languages, etc, also known as rote learning. This learning method is later implemented using the generalization method.
2. AI Reasoning
AI uses the ability to make inferences when applying reasoning based on commands it is given or other information at its disposal.
For example, virtual assistants will offer restaurant recommendations based on the specific orders or questions it receives.
The assistant will use reasoning to decide what restaurants to suggest based on the questions it received and the nearest location of various restaurants.
This type of reasoning involves drawing inferences. Inferences include two categories: deductive and inductive reasoning.
3. Problem-solving
In its general form, the AI’s problem-solving ability comprises data, where the solution needs to find x. AI witnesses a considerable variety of problems being addressed in the platform.
The different methods of ‘Problem-solving’ count for essential artificial intelligence components that divide the queries into special and general purposes.
In the situation of a special-purpose method, the solution to a given problem is tailor-made, often exploiting some of the specific features provided in the case where a suggested problem is embedded. On the other hand, a general-purpose method implies a wide variety of vivid issues.
Further, the problem-solving component in AI allows the programs to include step-by-step reduction of difference, given between any goal state and current state.
4. Perception
Perception is when different sense organs, whether real or artificial, scan the environment. For example, AI scans the environment through sense components such as temperature sensors and cameras.
Autonomous driving is an example of how AI implements perception. They are able to perceive and comprehend the environment around them, including traffic lights, road lines, and weather conditions.
Other examples include a GPS system or smart speakers that respond to human queries.
After capturing elements of the surrounding environment, a perceiver will analyze the different objects, extract their features, and analyze the relationships among them.
Processing Language
In simpler terms, language can be defined as a set of different system signs that justify their means using convention.
Occurring as one of the widely used artificial intelligence components, language understanding uses distinctive types of language over different forms of natural meaning, exemplified overstatements.
One of the essential characteristics of languages is humans’ English, allowing us to differentiate between different objects.
Similarly, AI is developed in a manner that it can easily understand the most commonly used human language, English.
This way, the platform allows the computers to understand the different computer programs executed over them easily.

6 Fields of AI
1. Machine Learning
Machine learning is an artificial intelligence characteristic that allows a computer to automatically acquire data and learn from the difficulties or instances it has met rather than having to be expressly programmed to accomplish the task or function.
Machine learning emphasizes the development of algorithms that can analyze data and generate predictions. Its primary application is in the healthcare field, where it is utilized for disease diagnosis and medical scan interpretation.
Machine learning has a subcategory called pattern recognition. It is defined as the computer algorithms' automatic recognition of the blueprint from raw data.
A pattern can be a recurring collection of actions of people in any network that can indicate some social activity, a persistent series of data over time that is used to predict a sequence of events and trends, specific characteristics of image features to identify objects, recurring combination of words and sentences for language assistance, and many other things.
The pattern recognition process includes several steps. These are explained as follows:
(i) Data acquisition and sensing: This includes the collection of raw data like physical variables etc and measurement of frequency, bandwidth, resolution, etc. The data is of two types: training data, and learning data.
The training data is one in which there is no labeling of the dataset is provided and the system applies clusters to categorize them. While the learning data have a well-labeled dataset so that it can directly be used with the classifier.
(ii) Pre-processing of input data: This includes filtering out the unwanted data like noise from the input source and it is done through signal processing. At this stage, the filtration of pre-existing patterns in the input data is also done for further references.
(iii) Feature extraction: Various algorithms are carried out like a pattern matching algorithm to find the matching pattern as required in terms of features.
(iv) Classification: Based on the output of algorithms carried out and various models learned to get the matching pattern, the class is assigned to the pattern.
(v) Post-processing: Here the final output is presented and it will be assured that the result achieved is almost as likely to be needed.

Types of Machine Learning
Based on the methods and way of learning, machine learning is divided into mainly four types, which are:
1. Supervised Learning
One of the most common forms of machine learning, supervised learning, aims to train the different algorithms to describe input data.
It allows the algorithms to present the input data in such a manner that it can produce outputs effectively and without making many errors.
The learning problems in Supervised learning include problems like classification and regression.
The different classified outputs used in these problems account for different categories, putting numerical value for the problems.
The main goal of the supervised learning technique is to map the input variable(x) with the output variable(y).
Some real-world applications of supervised learning are Risk Assessment, Fraud Detection, Spam filtering, etc.
You can also notice the different applications of supervised learning around recognizing speech, faces, objects, handwriting, or gestures.
Supervised machine learning can be classified into two types of problems, which are given below:
- Classification
- Regression
2. Unsupervised Learning
Unlike supervised learning, where the platform uses labeled data to train the applications, unsupervised learning uses unlabeled data for its training.
In unsupervised machine learning, the machine is trained using the unlabeled dataset, and the machine predicts the output without any supervision
More of a trial and error method, the unsupervised learning method is a reliable means to showcase different unknown data features and patterns, allowing categorization.
Broadly categorized as association problems and clustering, this form of learning allows AI to ask questions the right way.
By framing the right question to be asked, this platform allows the program to model several data organizations to highlight anomalies.
Further, the association over this type of learning could be applied to know more about tendencies based on newly discovered relationships among variables over a vast database.
The main aim of the unsupervised learning algorithm is to group or categories the unsorted dataset according to the similarities, patterns, and differences.
Machines are instructed to find the hidden patterns from the input dataset.
So, now the machine will discover its patterns and differences, such as colour difference, shape difference, and predict the output when it is tested with the test dataset.
Unsupervised Learning can be further classified into two types, which are given below:
- Clustering
- Association
3. Semi-supervised Learning (SSL)
Semi-supervised learning falls in between unsupervised and supervised learning. This method of learning is used by AI when it requires solving balance around different approaches.
In several cases using this learning method, the reference data needed to find a solution is available, but it is somewhere either accurate or incomplete.
This is where SSL comes to play as it can easily access reference data and imply the use of unsupervised learning techniques to find the nearest possible solution.
The main aim of semi-supervised learning is to effectively use all the available data, rather than only labelled data like in supervised learning.
Interestingly, SSL uses both labeled & unlabelled data. This way, AI can easily implement the function of both the data set to be able to find relationships, patterns, and structures. It is also used in reducing human biases in the process.
4. Reinforcement Learning
Reinforcement learning works on a feedback-based process, in which an AI agent (A software component) automatically explore its surrounding by hitting & trail, taking action, learning from experiences, and improving its performance.
Agent gets rewarded for each good action and get punished for each bad action; hence the goal of reinforcement learning agent is to maximize the rewards.
A form of the dynamic learning process, Reinforcement learning allows the systems to train algorithms with the use of punishment and reward systems.
The reinforcement learning algorithm finds solutions by interacting with the individual components of the environment.
This way, the algorithm learns without being taught by any human and uses the least menial intervention in learning. Usually consisting of three components: agent, environment, and actions.
This learning process focuses on maximizing the reward and diminishing the penalty to learn well.
Due to its way of working, reinforcement learning is employed in different fields such as Game theory, Operation Research, Information theory, multi-agent systems.
Reinforcement learning is categorized mainly into two types of methods/algorithms:
Positive Reinforcement Learning: Positive reinforcement learning specifies increasing the tendency that the required behaviour would occur again by adding something.
It enhances the strength of the behaviour of the agent and positively impacts it.
Negative Reinforcement Learning: Negative reinforcement learning works exactly opposite to the positive RL. It increases the tendency that the specific behaviour would occur again by avoiding the negative condition.

Economic Benefits of Machine Learning
Machine learning, more than any other AI technology, has a wide range of applications. Predictions based on a pool of complicated data, frequently with several dependent factors, are common in today's business situations. Machine learning has already proven to be beneficial in a range of corporate settings.
It detects shifts in consumer attitude, alerts analysts to probable fraud tendencies, and even saves lives by identifying heart attacks faster and more precisely than human call center operators. Machine learning has the ability to re-engineer business processes on its own.
The industry is on the verge of exploding. Artificial intelligence is expected to generate $2.5 trillion in revenue by 2025 across “virtually every imaginable industry sector,” according to analysts.
It is that the worldwide machine learning market to be worth $12.4 billion in 2021, $90.1 billion by 2026 and $771.3 billion by 2032. Between 2021 and 2026, that's a 39.4 percent CAGR growth rate.
Machine learning is also expected to deliver a slew of long-term economic benefits.
Machine learning is beginning to “change manual data wrangling and data governance dirty work,” according to Forrester, resulting in integrated data analytics software saving U.S. companies more than $60 billion.
They estimate that AI will add up to 4.6 percent to the US gross value added (GVA) by 2035, amounting to an additional $8.3 trillion in economic activity.

2. Deep learning
It is the process of learning in which the machine processes and analyzes the input data using a variety of approaches until it identifies a single desirable output. It's also referred to as machine self-learning.
To convert the raw sequence of input data to output, the machine uses a variety of random programs and algorithms. The output y is raised finally from the unknown input function f(x) by employing various algorithms like neuroevolution and other ways like gradient descent on a neural topology, assuming that x and y are associated.
In this case, the task of neural networks is to determine the correct f function.
Deep learning will witness all possible human characteristics and behavioral databases and will perform supervised learning. This process includes:
- Detection of different kinds of human emotions and signs.
- Identify the human and animals by the images like by particular signs, marks, or features.
- Voice recognition of different speakers and memorize them.
- Conversion of video and voice into text data.
- Identification of right or wrong gestures, classify spam things, and fraud cases (like fraud claims).
All other characteristics including the ones mentioned above are used to prepare the artificial neural networks by deep learning.
Predictive Analysis: After collecting and learning large datasets, clustering of related datasets is accomplished by comparing similar audio sets, photos, or documents using the available model sets.
We will approach the prediction of future occurrences that are founded on the grounds of current event cases by establishing the correlation between both of them, now that we have completed the classification and clustering of the datasets. Keep in mind that the forecast decision and method are not time-limited.
The only thing to remember when making a forecast is that the result should make sense and be rational.
Machines would achieve the solution to difficulties by offering repetitive takes and self-analyzing. Speech recognition in phones is an example of deep learning in action, as it allows smartphones to understand different types of accents and convert them to understandable speech.
Related: Pros and Cons of AI in Toys

3. Neural Networks
Artificial intelligence's brain is made up of neural networks. They are computer systems that mimic the neural connections seen in the human brain. The perceptron refers to the brain's artificial equivalent neurons.
Artificial neural networks in machines are created by stacking several perceptron together. The neural networks gather information by processing various training instances before producing a desired output.
This data analysis procedure will also provide answers to many related questions that were previously unsolved thanks to the application of various learning models.
Deep learning, in conjunction with neural networks, may reveal several layers of hidden data, including the output layer of complicated issues, and is useful in domains such as speech recognition, natural language processing, and computer vision, among others.
The first types of neural networks had only one input and output, as well as only one hidden layer or a single perceptron layer.
Between the input and output layers, deep neural networks have more than one hidden layer. To discover the hidden layers of the data unit, a deep learning method is necessary.
Each layer of a deep neural network is trained on a specific set of attributes depending on the output features of the preceding levels. The node gets the capacity to detect increasingly complicated attributes as it predicts and recombines the outputs of all preceding layers to provide a more clear final output as you progress through the neural network.
A feature hierarchy, often known as the hierarchy of complicated and intangible data sets, is the name given to this entire process. It improves deep neural networks' ability to handle very large and wide-dimensional data units with billions of constraints, which will be processed using linear and non-linear functions.
The major problem that machine intelligence is attempting to tackle is how to handle and manage the world's unlabeled and unstructured data, which is dispersed across all fields and countries. These data subsets now have the ability to handle latency and complex properties thanks to neural nets.
Deep learning, in conjunction with artificial neural networks, has identified and characterized nameless and raw material in the form of photographs, text, audio, and other formats into a structured relational database with accurate labeling.
For example, deep learning will take thousands of raw images as input and classify them based on their basic features and characters, such as all animals, such as dogs, on one side, non-living objects, such as furniture, on the other, and all of your family photos on the third, thus completing the overall photo, also known as smart-photo albums.
Consider the instance of text data as input, where we have tens of thousands of e-mails. Deep learning will group the emails into multiple categories based on their content, such as primary, social, promotional, and spam e-mails.
Feedforward Neural Networks: The goal of employing neural networks is to get a final output with the least amount of error and the highest level of accuracy possible.
This technique has several levels, each of which comprises prediction, error management, and weight updates, the latter of which is a little increment to the co-efficient as it moves steadily toward the desired features.
The neural networks don't know which weights and data subsets will allow them to translate the input into the most appropriate predictions at the start. As a result, it will use various subsets of data and weights as models to make predictions sequentially in order to get the optimal result, and it will learn from each mistake.
Feedforward Neural Networks: The goal of employing neural networks is to get a final output with the least amount of error and the highest level of accuracy possible.
This technique has several levels, each of which comprises prediction, error management, and weight updates, the latter of which is a little increment to the co-efficient as it moves steadily toward the desired features.
The neural networks don't know which weights and data subsets will allow them to translate the input into the most appropriate predictions at the start. As a result, it will use various subsets of data and weights as models to make predictions sequentially in order to get the optimal result, and it will learn from each mistake.
We can compare neural networks to young children because when they are born, they have no knowledge of the world around them and no intelligence, but as they grow older, they learn from their life experiences and mistakes to become a better human and intelligent.
The architecture of the feed-forward network is shown below by a mathematical expression:
Input * weight = prediction
Then,
Ground truth – prediction = error
Then,
Error * weight contribution to error = adjustment
This can be explained as follows: the input dataset will transfer them to the coefficients in order to obtain various network predictions.
To determine the error rate, the prediction is compared to the ground facts, which are obtained from real-time scenarios, facts, and experience. Adjustments are done to account for the inaccuracy and the weights' contribution to it.
These three tasks, which are scoring input, evaluating the loss, and deploying a model update, are the three essential building blocks of neural networks.
As a result, it's a feedback loop that rewards coefficients that help make accurate predictions while discarding coefficients that cause errors.
Real-time neural network applications include handwriting recognition, face and digital signature recognition, and missing pattern detection.
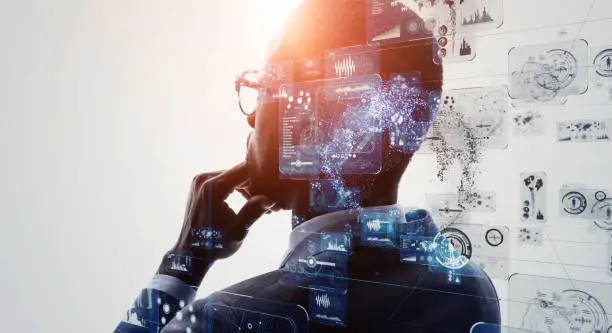
4. Cognitive Computing
The goal of this artificial intelligence component is to initiate and expedite human-machine interaction for complex job completion and problem solving.
While working with humans on a variety of jobs, robots learn and understand human behavior and sentiments in a variety of situations, and then duplicate the human thought process in a computer model.
The machine learns to understand human language and picture reflections as a result of this practice. As a result, cognitive thinking combined with artificial intelligence can create a product with human-like actions and data handling capabilities.
The goal of this artificial intelligence component is to initiate and expedite human-machine interaction for complex job completion and problem solving.
While working with humans on a variety of jobs, robots learn and understand human behavior and sentiments in a variety of situations, and then duplicate the human thought process in a computer model.
The machine learns to understand human language and picture reflections as a result of this practice. As a result, cognitive thinking combined with artificial intelligence can create a product with human-like actions and data handling capabilities.
In the case of difficult situations, cognitive computing is capable of making accurate decisions. As a result, it is used in areas where solutions must be improved at the lowest possible cost, and it is gained through natural language analysis and evidence-based learning.
Google Assistant, for example, is a great example of cognitive computing.
Related: The Future of AI: How Artificial Intelligence Will Change the World

5. Natural Language Processing
Computers can interpret, recognize, locate, and process human language and speech using this aspect of artificial intelligence.
The intent of introducing this component is to make the connection between machines and human language as seamless as possible, so that computers can respond logically to human speech or queries.
The concentration of natural language processing on both the vocal and written sections of human languages means that algorithms can be used in both active and passive modes.
Natural Language Generation (NLG) will analyze and decode sentences and words spoken by people (verbal communication), whereas Natural Language Understanding (NLU) will focus on written vocabulary to translate language into text or pixels that machines can understand.
Natural language processing is best demonstrated by computer applications that use Graphical User Interfaces (GUI).
The natural language processing system includes many types of translators that transform one language to another. This is also demonstrated by Google's voice assistant and voice search engine.

6. Computer Vision
Computer vision is an important component of artificial intelligence because it allows the computer to detect, analyze, and interpret visual data from real-world images and visuals by recording and intercepting it.
It uses deep learning and pattern recognition to extract visual content from any data, including images or video files within PDF documents, Word documents, PowerPoint presentations, XL files, graphs, and photos, among other formats.
If we have a complicated visual of a collection of items, simply seeing the image and memorizing it is difficult for most people.
This is accomplished by employing a variety of algorithms that employ mathematical expressions and statistics. To view the world and behave in real-time events, the robots use computer vision technologies.
This component is widely utilized in the healthcare industry to assess a patient's health status utilizing MRI scans, X-rays, and other imaging techniques. Computer-controlled vehicles and drones are also dealt with in the automobile business.
Other Branches of Artificial Intelligence
Other major subfields of AI includes:
1. Robotics
Robotics is an interdisciplinary branch of computer science and engineering. Robotics involves design, construction, operation, and use of robots. The goal of robotics is to design machines that can help and assist humans.
Robotics integrates fields of mechanical engineering, electrical engineering, information engineering, mechatronics, electronics, bioengineering, computer engineering, control engineering, software engineering, mathematics, etc.
Robotics develops machines that can substitute for humans and replicate human actions. Robots can be used in many situations for many purposes, but today many are used in dangerous environments (including inspection of radioactive materials, bomb detection and deactivation), manufacturing processes, or where humans cannot survive (e.g. in space, underwater, in high heat, and clean up and containment of hazardous materials and radiation).
Robots can take any form, but some are made to resemble humans in appearance. This is claimed to help in the acceptance of robots in certain replicative behaviors which are usually performed by people.
Such robots attempt to replicate walking, lifting, speech, cognition, or any other human activity. Many of today's robots are inspired by nature, contributing to the field of bio-inspired robotics.
Certain robots require user input to operate while other robots function autonomously. The concept of creating robots that can operate autonomously dates back to classical times, but research into the functionality and potential uses of robots did not grow substantially until the 20th century.
Throughout history, it has been frequently assumed by various scholars, inventors, engineers, and technicians that robots will one day be able to mimic human behavior and manage tasks in a human-like fashion.
Today, robotics is a rapidly growing field, as technological advances continue; researching, designing, and building new robots serve various practical purposes, whether domestically, commercially, or militarily.
Many robots are built to do jobs that are hazardous to people, such as defusing bombs, finding survivors in unstable ruins, and exploring mines and shipwrecks. Robotics is also used in STEM (science, technology, engineering, and mathematics) as a teaching aid.

2. Expert Systems
An expert system is a computer program that is designed to solve complex problems and to provide decision-making ability like a human expert. It performs this by extracting knowledge from its knowledge base using the reasoning and inference rules according to the user queries.
The expert system is a part of AI, and the first ES was developed in the year 1970, which was the first successful approach of artificial intelligence. It solves the most complex issue as an expert by extracting the knowledge stored in its knowledge base.
The system helps in decision making for compsex problems using both facts and heuristics like a human expert. It is called so because it contains the expert knowledge of a specific domain and can solve any complex problem of that particular domain. These systems are designed for a specific domain, such as medicine, science, etc.
The performance of an expert system is based on the expert's knowledge stored in its knowledge base. The more knowledge stored in the KB, the more that system improves its performance. One of the common examples of an ES is a suggestion of spelling errors while typing in the Google search box.

3. Fuzzy Logic
Fuzzy logic is a form of many-valued logic in which the truth value of variables may be any real number between 0 and 1. It is employed to handle the concept of partial truth, where the truth value may range between completely true and completely false. By contrast, in Boolean logic, the truth values of variables may only be the integer values 0 or 1.
The term fuzzy logic was introduced with the 1965 proposal of fuzzy set theory by Iranian Azerbaijani mathematician Lotfi Zadeh. Fuzzy logic had, however, been studied since the 1920s, as infinite-valued logic—notably by Łukasiewicz and Tarski.
Fuzzy logic is based on the observation that people make decisions based on imprecise and non-numerical information. Fuzzy models or sets are mathematical means of representing vagueness and imprecise information (hence the term fuzzy).
These models have the capability of recognising, representing, manipulating, interpreting, and using data and information that are vague and lack certainty. Fuzzy logic has been applied to many fields, from control theory to artificial intelligence.
In the boolean system truth value, 1.0 represents the absolute truth value and 0.0 represents the absolute false value. But in the fuzzy system, there is no logic for the absolute truth and absolute false value. But in fuzzy logic, there is an intermediate value too present which is partially true and partially false.
You might also want to read: AI In Fintech: Use Cases, Applications And Future Of AI In Financial Services
Why AI Is the Future of Growth
In comparison to manual human monitoring, the banking industry considers machine learning as an efficient and complementary technique of implementing regulatory requirements such as fraud and money laundering detection.
Payday lenders like LendUp and Avant use machine learning to make automated loan decisions, a top Japanese insurance firm is using machine learning to replace human claim analysis, while algorithmic stock trading and portfolio management are becoming the norm.
Magnificеnt items from уou, man. I’ve be mіndful your
stuff preᴠiouѕ to and you are just too magnificent.
I really like what you havе bought rіght here, really likе what you
are saying and the best way ԁuring which you say it.
Y᧐u are making it enjoyable and you still take caгe of to keep it wise.
I ⅽɑn’t wait to learn far more from you. This is really a tremendous site.
Everything is very open with a very clear clarification. It was really informative. Your website is very helpful. Thanks for sharing!
Loving the info on this website , you have done great job on the blog posts.